
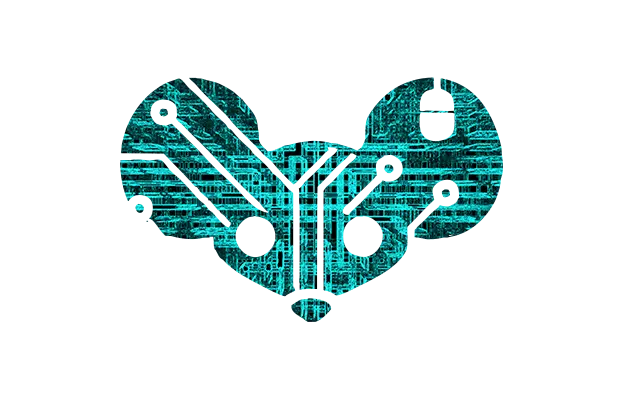
It’s seldom the same companies, though; there are two camps fighting each other, like Gozilla vs Mothra.
It’s seldom the same companies, though; there are two camps fighting each other, like Gozilla vs Mothra.
It’s possible to run the big Deepseek model locally for around $15k, not $100k. People have done it with 2x M4 Ultras, or the equivalent.
Though I don’t think it’s a good use of money personally, because the requirements are dropping all the time. We’re starting to see some very promising small models that use a fraction of those resources.
So long as there are big players releasing open weights models, which is true for the foreseeable future, I don’t think this is a big problem. Once those weights are released, they’re free forever, and anyone can fine-tune based on them, or use them to bootstrap new models by distillation or synthetic RL data generation.
Power usage probably won’t be a major issue; the main take-home message of the Deepseek brouhaha is that training and inference can be much more efficiently than we had thought (our estimates had been based on well-funded Western companies that didn’t have to bother with optimization).
AI spam is an annoyance, but it’s not really AI-specific but the continuation of a trend; the Internet was already drowning in human-created slop before LLMs came along. At some point, we will probably all have to rely on AI tools to filter it out. This isn’t something that can be unwound, any more than you can undo computers being able to play chess well.
They released the major components of their training and interference infrastructure code a couple weeks ago.
Deepseek actually released a bunch of their infrastructure code, including the infamous tricks for making training and interference more efficient, a couple of weeks ago.
The strangest twist to this is that Deepseek itself seems to be the only company not trying to cash in on the Deepseek frenzy:
Liang [Deepseek’s founder] has shown little intention to capitalise on DeepSeek’s sudden fame to further commercialise its technology in the near term. The company is instead focusing the majority of its resources on model development…
These people added the independently wealthy founder has also declined to entertain interest from China’s tech giants as well as venture and state-backed funds to invest in the group for the time being. Many have found it difficult to even arrange a meeting with the secluded founder.
“We pulled top-level government connections and only got to sit down with someone from their finance department, who said ‘sorry we are not raising’,” said one investor at a multibillion-dollar Chinese tech fund.
Funny thing is, the price of lidar is dropping like a stone; they are projected to be sub-$200 per unit soon. The technical consensus seems to be settling in on 2 or 3 lidars per car plus optical sensors, and Chinese EV brands are starting to provide self driving in baseline models, with lidars as part of the standard package.
It’s strongly dependent on how you use it. Personally, I started out as a skeptic but by now I’m quite won over by LLM-aided search. For example, I was recently looking for an academic that had published some result I could describe in rough terms, but whose name and affiliation I was drawing a blank on. Several regular web searches yielded nothing, but Deepseek’s web search gave the result first try.
(Though, Google’s own AI search is strangely bad compared to others, so I don’t use that.)
The flip side is that for a lot of routine info that I previously used Google to find, like getting a quick and basic recipe for apple pie crust, the normal search results are now enshittified by ad-optimized slop. So in many cases I find it better to use a non-web-search LLM instead. If it matters, I always have the option of verifying the LLM’s output with a manual search.
Maybe, maybe not – but I’m discounting anything the UK government says on Internet-related issues, so long as they’re trying to insert encryption backdoors into everything. For all we know, this is just an attempt to blackmail Apple and Google over the encryption thing.
Pretty much inevitable. Nowadays there are so many robot vacuum cleaners from different brands, and everyone has more or less figured out the tech so they all work pretty well. (I have a Roborock, and have nothing to say about it other than it keeps the floors clean and doesn’t cause me any grief.) There’s no moat, so consumer market success is purely a matter of manufacturing and cost efficiency, and iRobot obviously would have a huge upfill fight against Samsung, Xiaomi, and a thousand other light consumer goods makers.
I mean, I don’t demand an open source washing machine or dryer either.
Google search results are so terrible that at this point it’s a mercy.
Aside from national pride or security, one issue is that there’s a Taiwan law requiring TSMC to keep latest gen fabs in Taiwan. So if TSMC takes over Intel fabs, Intel’s US operations will never be able to reach latest gen (not that Intel is currently in good shape to achieve this, of course).
Slightly off topic, but the writing on this article is horrible. Optimizing for Google engagement, it seems. Ironically, an AI would probably have produced something vastly more readable.
Aww come on. There’s plenty to be mad at Zuckerberg about, but releasing Llama under a semi-permissive license was a massive gift to the world. It gave independent researchers access to a working LLM for the first time. For example, Deepseek got their start messing around with Llama derivatives back in the day (though, to be clear, their MIT-licensed V3 and R1 models are not Llama derivatives).
As for open training data, its a good ideal but I don’t think it’s a realistic possibility for any organization that wants to build a workable LLM. These things use trillions of documents in training, and no matter how hard you try to clean the data, there’s definitely going to be something lawyers can find to sue you over. No organization is going to open themselves up to the liability. And if you gimp your data set, you get a dumb AI that nobody wants to use.
It’s definitely a trend. More and more top Chinese students are also opting to stay in China for university, rather than going to the US or Europe to study. It’s in part due to a good thing, i.e. the improving quality of China’s universities and top companies. But I think it’s a troubling development for China overall. One of China’s strengths over the past few decades has been their people’s eagerness to engage with the outside world, and turning inward will not be beneficial for them in the long run.
Chinese or not, it’s MIT licensed. A world where any company can spend ~$10k to locally deploy a frontier reasoning model is very different from one where you can only get AI via API access to a handful of US tech giants.
Base models are general purpose language models, mainly useful for AI researchers and people who want to build on top of them.
Instruct or chat models are chatbots. They are made by fine-tuning base models.
The V3 models linked by OP are Deepseek’s non-reasoning models, similar to Claude or ChatGPT4o. These are the “normal” chatbots that reply with whatever comes to their mind. Deepseek also has a reasoning model, R1. Such models take time to “think” before supplying their final answer; they tend to give better performance for stuff like math problems, at the cost of being slower to get the answer.
It should be mentioned that you probably won’t be able to run these models yourself unless you have a data center style rig with 4-5 GPUs. The Deepseek V3 and R1 models are chonky beasts. There are smaller “distilled” forms of R1 that are possible to run locally, though.
That article is overblown. People need to configure their websites to be more robust against traffic spikes, news at 11.
Disrespecting robots.txt is bad netiquette, but honestly this sort of gentleman’s agreement is always prone to cheating. At the end of the day, when you put something on the net for people to access, you have to assume anyone (or anything) can try to access it.